KEYNOTE SPEAKERS
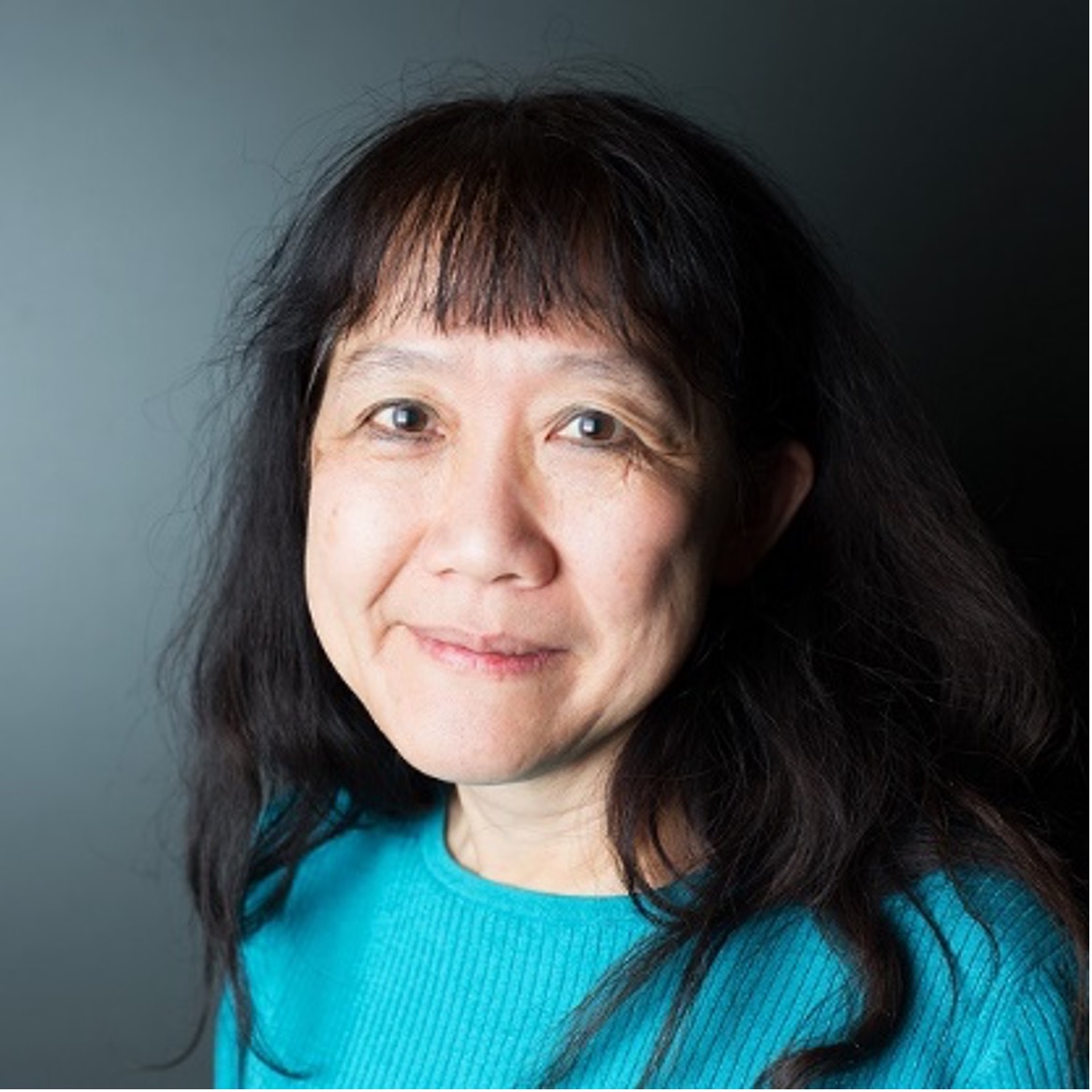
Shih-Chii Liu
Institute of Neuroinformatics, University of Zurich and ETH Zurich
Bringing dynamic sparsity to low-power edge computing
Abstract:
Dynamic sparsity is intrinsic to biological computing and is key to its extreme power efficiency. Edge computing systems can improve their energy efficiency and reduce response latency by exploiting this neuromorphic principle. In edge computing, the neuromorphic approach for extracting features replaces conventional ADC and DSP with biological-inspired filters and event generators implemented in mixed-signal circuits. The resulting sparse feature events drive inference in dynamic-sparsity-aware neural network accelerators to reduce computational load and memory access. The demonstration of an ASIC combining the feature extractor and postprocessing network in an edge audio task shows the dynamic savings in power. Exploiting dynamic sparsity at all levels will benefit the design of intelligent devices for edge, wearable and biomedical applications.
Biography:
Shih-Chii Liu received the BS degree in electrical engineering from MIT and the PhD degree in the Computation and Neural Systems program from Caltech. She is Adjunct Professor in the Faculty of Science at the University of Zurich, Switzerland. She co-directs the Sensors group (http://sensors.ini.uzh.ch) at the Institute of Neuroinformatics, University of Zurich and ETH Zurich. Her group works on ASIC design of low-power neuromorphic auditory sensors, event-driven bio-inspired processing models and deep neural network algorithms; and the use of these networks in neuromorphic artificial intelligent systems. Dr. Liu is past Chair of the IEEE CAS Sensory Systems and Neural Systems and Applications Technical Committees. She is past associate editor of the IEEE Transactions of Biomedical Circuits and Systems and Neural Networks journal. She was the general co-chair of the 2020 IEEE International Conference on Artificial Intelligence Circuits and Systems (AICAS) and a technical committee member of 2023 IEEE AICAS. She has been current Chair of the IEEE Swiss CAS/ED Society and is a technical committee member of 2025 IEEE Custom Integrated Circuits Conference (CICC).
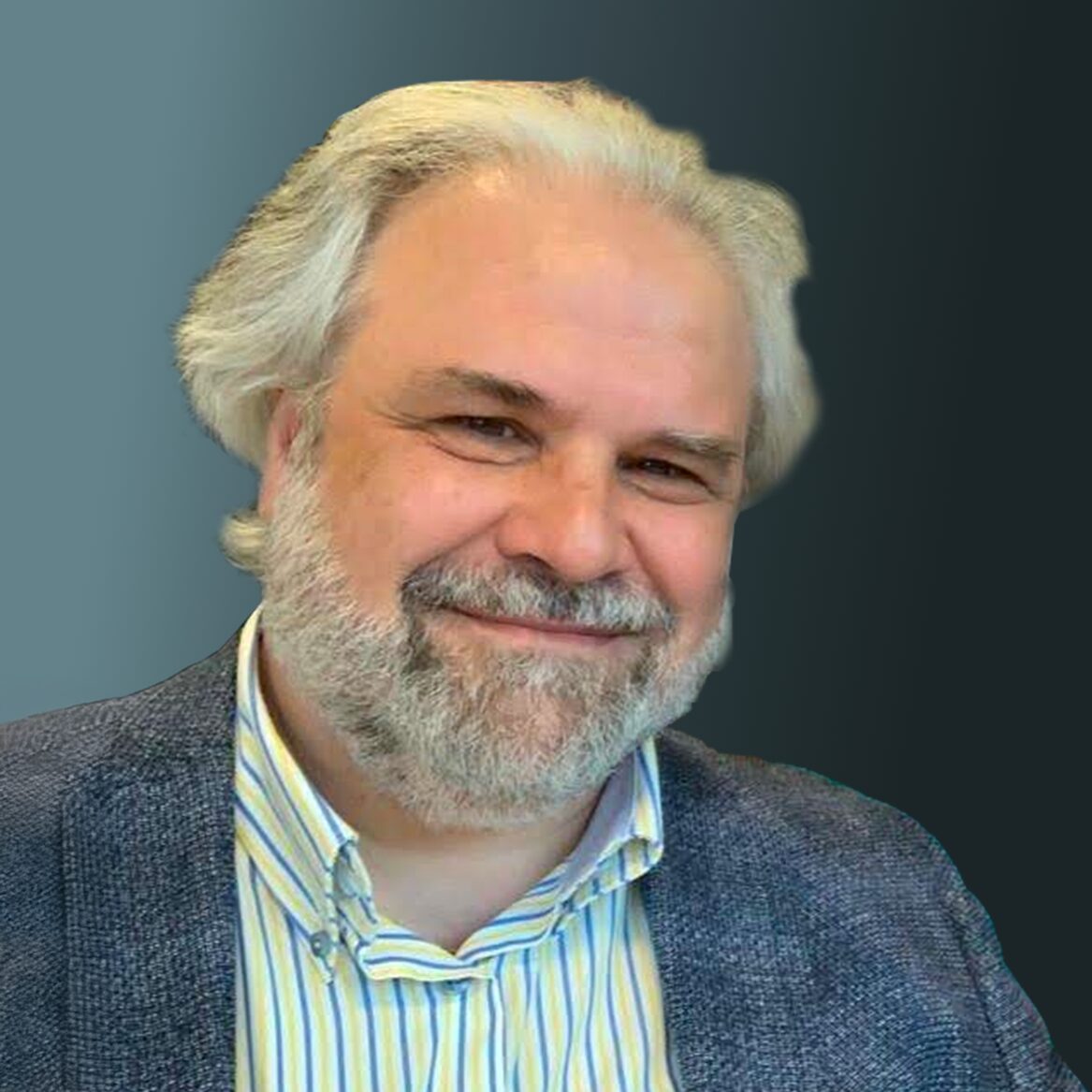
Giuseppe Desoli
PhD, STMicroelectronics Company Fellow
Senior Director of Artificial Intelligence & Embedded Architectures
Pushing the Boundaries of Edge AI: The Potential and Challenges of In-Memory Computing Technologies
Abstract:
In-memory computing (IMC) can significantly enhance tasks such as matrix-vector multiplication in edge AI algorithms. Despite its promises, IMC faces substantial challenges due to device and circuit non-idealities. The state-of-the-art spans a wide range of device technologies and diverse digital, analog, and mixed design approaches. Non-volatile and emerging memories are pivotal for integrating IMC into various products and edge AI applications, addressing energy and compute density constraints.
With the advent of large language models (LLMs) like GPT-4, only some 3D stacked technologies hold the potential to support the required scale. At the edge, traditional Von Neumann architectures, near-memory, and in-memory computing approaches each offer unique benefits and challenges. Energy efficiency varies significantly between SRAM IMC and purely digital architectures, with future process and technology advancements potentially narrowing this gap. Crosscutting issues such as on-device learning, quantization, security, and error correction highlight the complexity of IMC technologies. Beyond machine learning, IMC shows promise in hyperdimensional computing, associative memories, and probabilistic computing. Additionally, the ability of software mapping tools to efficiently adapt high-level algorithmic representations for edge AI is a crucial component of the overall picture.
While emerging memory AI solutions through IMC are not yet competitive with SRAM IMC, emerging optimizations and technologies hold disruptive potential. Edge AI algorithms continue to evolve, and hybrid approaches remain essential for edge AI hardware acceleration.
Biography:
Giuseppe Desoli holds a Master and PhD in Electrical Engineering from the University of Genoa. From 1995 to 2002, he worked at Hewlett-Packard Laboratories, developing VLIW microprocessor architectures, compilers, and tools. In 2002 joined STMicroelectronics as an R&D Director and lead microprocessor architect, pioneering multiprocessor systems for embedded SoCs. Since 2012, he is serving as Company Fellow and Chief Architect for the System Research & Application central R&D group, he is the chairman of the ST scientific committee and coordinator for the ST’s Artificial Intelligence Affinity Team, leading the development of different technologies in the field of HW accelerated AI and In-Memory-computing for advanced deep learning applications. He has co-authored over 70 scientific publications and holds more than 60 patents.